From predicting extreme events to helping policy makers, AI promises endless application on paper, but a closer inspection of the technology reveals significant wrinkles
History is occasionally punctuated by intervals of time that accelerate humanity’s march towards the future. If media coverage is to be taken at face value, we are currently in such an exponential phase. Artificial intelligence has well and truly brimmed over the cauldron of public imagination and is uncontrollably spilling over into the realm of reality.
Naturally, with the unprecedented ability to analyse colossal datasets on offer, scientists studying climate change and feedback in the earth system have eyed the potential of AI for a while now. The incredible computational capacity and automated iterative learning ability brought forth by machine learning are now increasingly finding use among industry, particularly in the energy sector, to optimise operations and reduce wastages. The case for the application of AI to climate action seems to be an intuitive one. The implications, however, could be far wider than the intent would suggest.
Flavour of the season
Recent developments in AI technology have not come out of the blue. Early theorists of AI date back to the middle of the 20th century, but it wasn’t until the proliferation of the internet that the concept became widely accepted. AI essentially refers to a kind of information and communication technology that has the ability to mimic human intelligence by combing datasets for patterns and rules unspecified by the programmer and optimising the output. All in all, such intelligent machine systems display “a combination of classification, prediction, and decision-making.” Machine learning (ML) and deep learning, which are essentially learning methods covered under the broad term AI, leverage computational networks called neural networks and algorithmic models to analyse structured datasets such as rainfall information and unstructured data such as raw images. The idea is to optimise results to an extent that has been thus far impractical under conventional methods of analysis.
The application of AI in the climate change arena can broadly be traced under three categories—data analysis, process optimisation and scenario modelling. The promise of AI and ML in being able to scour inhibitively large datasets, identify previously unknown trends and optimise for efficiency in novel ways naturally finds immense utility in climate change research and climate action, and is unsurprisingly one of the most active areas of AI research and development.
This ability has already been widely used to unwind multi-dimensional climate datasets and for the forecasting of future trends. One such model found wide coverage in the news in the past week after it revealed that earth is on the brink of crossing the 1.5°C threshold of the Paris Agreement and would likely cross it within this decade. Similar models are being used to track and forecast temperature trends, oceanic and atmospheric circulations, complex weather systems and societal responses such as migration. ML models have enabled automated and continuous monitoring of satellite images to pick out and report visible phenomena such as wildfires, landslides and deforestation through pattern and image recognition. Not only can AI tools be used to anticipate extreme weather events that have become more likely due to the effects of climate change, recent developments have shown how AI can be used to anticipate and plan for knock-on effects of such events.
To cite just one example, researchers have shown how AI tools can be integrated into agricultural weather advisories to optimise water use on farms. Piloting the model on grape farms in Nashik, researchers from IIT-Bombay, Indian Institute of Tropical Meteorology and Sensartics Pvt. Ltd have claimed water savings between 17% and 45% depending on the time of the year.
Similar utility has been demonstrated in the area of climate change mitigation as well. A 2018 paper produced by Microsoft and PwC estimates that the AI application for environmental objectives under a business-as-usual scenario could boost global GDP by up to 4.4% while reducing GHG emissions anywhere between 1.5% and 4% by the end of this decade. And proof of this potential is abundant in the applications that have been developed so far, with AI tools having been developed to maximise generation from renewable energy installations as well as increase energy efficiency in heavy industry, particularly in the petro-chemical sector. Other applications have explored sectoral and industry-level emission optimisation—huge in terms of potential mitigation of GHG emissions. Examples include but are far from being limited to the monitoring and reduction of emission intensity of industrial production processes and construction activity, increasing energy efficiency of transport, public infrastructure and buildings, and the optimisation of electric grid operations. At a larger scale, the technology at present is also being utilised to study carbon removal from the atmosphere and plan for future sequestration needs.
AI approaches are also being designed to aid policy-making for effective climate action with tools offering predictive insights to roll out policy interventions such as carbon tax regimes and carbon trading mechanisms. Beyond statistical assessments, the tools on offer through AI could be deployed for qualitative sentiment analysis for effective granular and localised policy making.
Unsurprisingly, interest in leveraging the technology has not remained limited to academic circles. Recent trends show a clear and definitive growth in the application of AI in commercial enterprises. An assessment of over six million US patents between 1979 and 2019 shows an exponential growth in the patents granted in the intersection of climate and AI, although still forming only a small fraction of the total patents granted individually in the spaces of climate and AI. The analysis found most common areas of application to be transportation, energy and industrial production technologies.
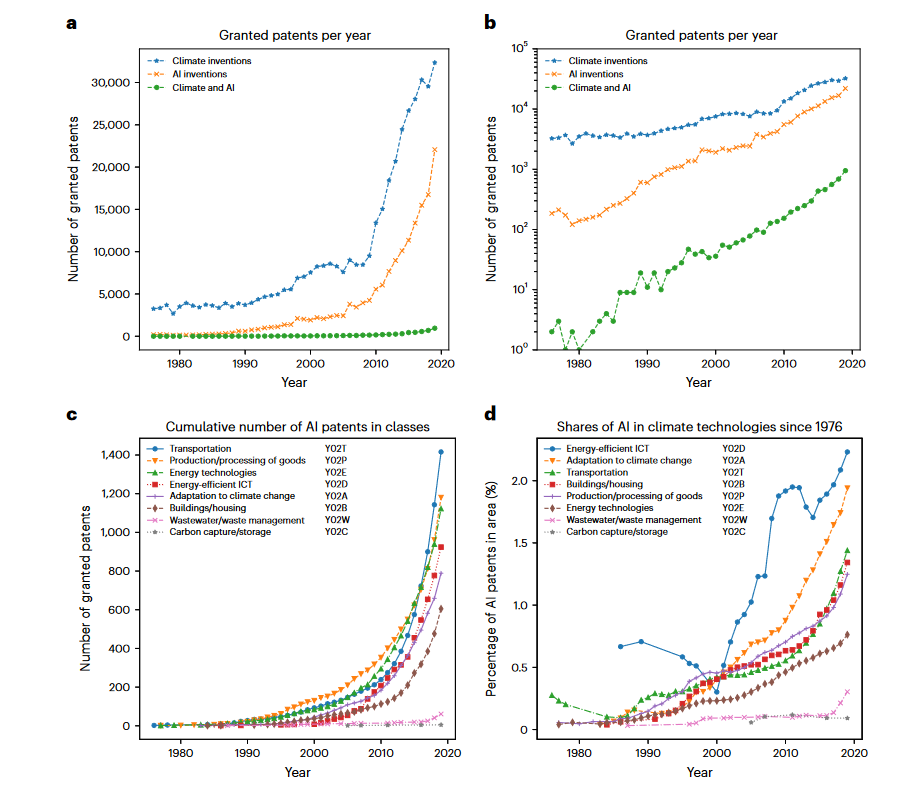
Given the seemingly endless spheres of application that AI promises on paper, it is easy to construe AI as some sort of a silver bullet. A closer inspection of the technology, however, reveals significant wrinkles.
Uncomfortable truths
AI models, for all their potential, have a pretty significant Achilles heel. Simulating intelligent and self-correcting actions while dealing with superlative, multi-dimensional datasets is not exactly energy efficient. As it happens, training an AI model and operating the infrastructure (read data centres) required to run these models carry significant energy demand and a growing carbon footprint. Despite advances in hardware efficiency providing significant emission reductions, AI models are far from having a clear negative impact on emissions. According to an assessment by the International Energy Agency in 2020, if efficiency improvements in hardware and data centre infrastructure can be sustained at the current rates, energy demand from the ICT sector “can remain nearly flat through 2022, despite a 60% increase in service demand.” A drastic drop in energy and emission intensity of the infrastructure has been shown through a renewable energy integration, improved cooling systems, efficient processors, heat recycling processes, and carbon offsetting. There is, however, little evidence that IEA’s prediction has materialised.
Probing methods to evaluate the net impact of AI models on emissions through applications, researchers studied emissions in three AI models applied on well-differentiated real-world conditions, parameters and requirements. The results, published last month, found that when both positive and negative impacts on emissions are considered, models sometimes added more emissions than they removed. Interestingly, as models were scaled up to include more users, the net effect was once found to be positive in terms of emission reductions.
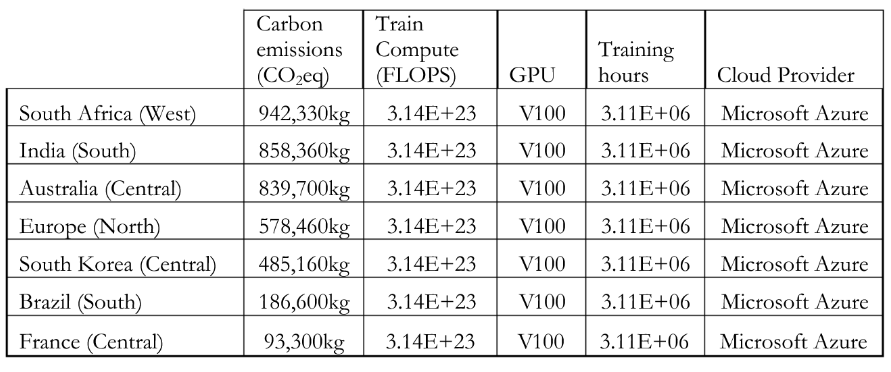
Emission profiles of AI models are not linear, and neither do they remain uniform across use cases. For data-driven ML-based research applications, like those used by researchers in the above study, the preparatory phase in which the model is trained is often more energy intensive. These models typically become more efficient as they are scaled. This inverse proportionality, however, does not hold true for AI applications in production systems, which become more energy intensive as they are scaled up.
By itself, increased efficiency of production processes does not guarantee net reductions in emissions either. While improved efficiency of production processes leads to lower energy, resource and capital inputs required per unit, such changes in the past have often resulted in increased demand and an overall increase in resource demand from the sector. Literature on the application of AI to climate action is replete with consideration of this “rebound effect,” most of which include warnings to governments looking to leverage the potential of AI for climate action and economic growth.
As AI gets integrated into the modelling of shared socioeconomic pathways (SSPs) for emission reductions, governments will also have to contend with its political implications. Calls have been growing for greater reflection of equity in mitigation pathways endorsed by the IPCC. Meanwhile, recent editions of the UNFCCC COP have been charged with fraught deliberations over the validity and scope of guiding principles of the UN climate convention. With AI thrown into the mix, these battles look set to intensify over the remainder of this decade. While the technology offers tools for more accurate representation of equity, doing so agnostically is likely to produce pathways that demand drastic and rapid reductions of emissions from developed countries—an untenable proposition politically for governments in these countries.
On the other hand, early developments are likely to be concentrated in developed economies, which can meet the requirements of the capital and resource intensive technology. Under the present dominant narratives that suggest the infallibility of AI, governments investing early in the intersection of AI and climate and energy modelling hold the intangible advantage of setting the baselines. Experience with energy and climate modelling in general certainly add weight to this possibility. The mere connection with AI carries an aura of credibility—and the risk of false credibility, which could very well be exploited to downplay inconvenient parameters (like those describing equity) and highlight favourable pathways.
Flashpoints ahead
These risks have not gone unnoticed. Neither has the immense potential the technology brings. Over the past year or so, attempts have been made to frame some guidelines and recommendations for governments to evaluate and assess the impact of AI in terms of energy demand and net effect on emissions. The guidelines, however, still remain broadly amorphous in their advocacy for effective governance structures and mechanisms for checks and balances.
Despite the ability to self-correct and evolve, AI still remains fundamentally agnostic. A realistic assessment of the potential of AI in tackling climate change is incomplete without recognising this fact. As any tool, the technology is ultimately only as useful as the brains that mould it design it to be. While AI applications undoubtedly hold the potential to revolutionise research, production processes and social interactions, unlocking this potential will finally depend on how the algorithm directing a model’s learning processes is composed.
It might take some time for the hype around AI to die down and for expectations to be tempered to be in more line with reality. It would be hardly a surprise if AI is ultimately leveraged for a net positive impact in the response to climate change when that happens. The journey to that point though will likely pass through multiple economic, political and ethical flashpoints.
About The Author
You may also like
India’s EV revolution: Are e-rickshaws leading the charge or stalling it?
Is pine the real ‘villain’ in the Uttarakhand forest fire saga?
NCQG’s new challenge: Show us the money
India’s energy sector: Ten years of progress, but in fits and starts
9 years after launch, India’s solar skill training scheme yet to find its place in the sun